AGI Icons S01E01
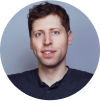
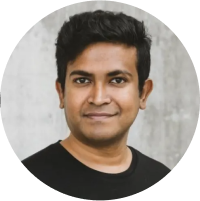
John:
All right, it’s great to be here. Is everyone excited?
Sam:
Yeah.
John:
Great, and before we start, and this is of course Turing’s first AGI Icons series. Quick show of hands, how many in the audience are working on research or engineering in AI? Okay, great. Do we have a good chunk of SF’s AI community here? That’s great. That’s awesome. And it’s my pleasure, thank you, Sam, for being here.
Sam:
Thanks for having me.
John:
It’s great to kick off our AGI Icons series with the company that kicked off the AGI era, OpenAI with, ChatGPT. Super exciting to do this with you, and everyone has access to the QR codes. You can ask questions there. Sam, for today’s conversation, I thought we’d talk about three things. First, OpenAI’s mission. Second, the state of AGI and where things are headed. And finally, the adoption of AGI inside businesses and what are some of the barriers holding things back.
So those would be sort of the three major themes that we’ll touch across. And we’re going to have lots of time for audience questions as well, so please make sure that you have time for that. Firstly, it’s just incredible to see San Francisco being at the heart of the AI revolution, and Sam, San Francisco has always been special to you. Why is that?
Sam:
I’ve lived here for a long time. I like it, in spite of… It’s been a tough time, but I think you don’t abandon good places, good friends, during tough times, and I’m very grateful that SF has existed, and I think the tech scene here and sort of the culture from here has been an important thing in the world to cherish. And I think right now it feels like Florence during the Renaissance or something. I mean, it feels like the center of the world, in a cool way.
John:
Yeah, it does feel like the center of the world in a cool way. A big round of applause for San Francisco. Great, great. And OpenAI, of, course is based in the mission. And speaking of mission, okay, this is a really bad segue, so out of all the companies that I’ve had an opportunity to observe, I felt like the company with the most inspiring mission I could think of is OpenAI. Sam, could you talk a little bit about OpenAI’s mission and why it’s been so critical to attracting great talent?
Sam:
I mean, I think if we are successful in building AGI and figuring out how to make it safe, and help people, and deploy it widely, and let people use it, there’s sort of no work required to make that an exciting mission. That will be one of the greatest quests in human history. That’ll be one of the most… I certainly cannot imagine a more fun, more exciting, more important thing to work on.
And I think, in addition to what that will do for the world, I think the prosperity that would come from truly abundant intelligence and the ability to do things beyond what humans can do on their own, it’s also just incredibly fun and special to be in the room on the forefront of scientific discovery. We get to see what’s going to happen a little bit before anybody else, and we get to figure out what I think is the most interesting puzzle I can imagine. And so that’s quite rewarding to work on.
John:
That’s great. Who here finds OpenAI’s mission inspiring? Okay, great. A lot. It is incredible, right? And one thing that you’ve shared before, Sam, that building a startup company is so hard, you might as well work on a really hard problem, and smart people gravitate towards hard problems. So it’s a win on attracting great talent and increasing your odds of success.
Sam:
Yeah, I mean, I think there’s a lot of things at play there. Definitely, any startup is going to be super hard, so it’s not that much harder, and in many ways, it’s easier to sort of go after something that’s going to take a long time. There are some tricks to it. Like, at the beginning, we had an attack vector for how we were going to build AI, but it’s hard to overstate how back in 2016, no one believed that AGI was a credible thing to go after.
And we kind of knew that we were going to push on a few things, but we certainly had not thought about language models at that point, in a serious way. So if you’re working on something very uncertain, which was like how to build AGI, we looked around the first day, we said, “We have no idea. We know that deep learning gets better with scale. We don’t really know what problem to work on. We don’t know what we can do now. We don’t know what we’re going to pursue as a project.” It’s remarkable how, if you could go back in time, how little we knew.
There’s this phenomenon, I don’t know a good phrase for it, it’s like it’s the novice’s edge. If you knew then what you know now, you would never try, because it seems too unlikely and too hard. And if we knew everything now, we wouldn’t have started OpenAI, but we did. And that’s important, and you just jump into things, and get a few lucky breaks, and figure out how to make it work.
But although the beginning part is harder, and certainly it was for us, we really had to figure out how we were going to come up with an AGI research program, and get any success, and had no idea about how much compute we’re going to need, or a product, or a business model, or anything. We didn’t launch our first product for four and a half years, so that period was tough. But then, conditioned on getting it to work, if it is something that is really exciting and transfixes people, everything else is easier.
John:
Great, and there clearly is something special about OpenAI. I mean, there is something different about the pace at which you ship. It feels like the company just operates on a different clock pulse. Why did something like ChatGPT, Sora, DALL·E come out of OpenAI in the past? I’ve just seen all of these research labs-
Sam:
I think we just care more. I mean, I think we just, we work really hard. We don’t have the cruft of a big company like a Google or something, but we really care about what we’re doing.
John:
And it seems like you think deeply about the intersection of product… Sorry, research, engineering and safety. Can you speak a little more-
Sam:
And the product. There have not been many great research labs in Silicon Valley in a long time, for whatever reason. Silicon Valley used to be really good at these things, and then since Xerox, PARC, maybe not as much as we should hold ourselves to. And when we were starting this, we said we couldn’t totally understand why, but we said, “We’re going to try really hard to learn about how to build a great research lab,” and we found some of those old people from PARC and elsewhere.
But we also said, “If this does work, we’re going to have to figure out how to make it the startup version of a research lab, because that’s what we understand.” And one of the things that we believed from the very beginning, more so even later, is that we were going to need to do research and engineering together. And some of the other AI research efforts that were doing that was really bad is they valued great research, but they didn’t value great engineering.
And so you had people thinking about these interesting ideas and these interesting papers, but you couldn’t implement them at scale. So we said, “Okay, we know how to build a good engineering team and a good engineering culture. We’re going to do that and a research culture together.” We had started with safety, and we really, really care about safety. So we said, “We also, we’re going to try our hardest to figure out to make these systems safe.” And we did those three things for a while, and then we realized we also were going to have to deploy AGI and build products, and so that was another thing.
And the thing, one of the most interesting and hardest challenges of the job has been figuring out how to build a culture that values all of those things, and it’s not there’s one first class citizen and everything else is sort of neglected. And where you get all of those different areas of expertise to work together towards one harmonious, “We care and we’re going to get the details right,” thing.
John:
That’s great, that’s great. I mean, we’ve had the privilege of working with OpenAI on a few things, and it never feels like we’re working with a big company. It feels like you’re working with another startup, because you [inaudible 00:08:57]-
Sam:
[inaudible 00:08:57], yeah.
John:
Yeah, great. So different people have different definitions of AGI. What is your definition of AGI?
Sam:
I don’t think it matters. I think it’s like a dumb term at this point, honestly. I think it basically means smarter systems than we have today that are coming at some point in the relatively approachable future. But it’s all just like we’re on one continuum of increasing intelligence. You can draw an X on that curve if you want, somewhere, and say, “This is the AGI point,” but there’s stuff before that point that’s really impactful. There’s stuff after that point that’ll be really impactful.
I think that the shift in perspective to it being a continuum, that matters, and the fact that the curve is going to keep going, not a point that the AGI is AGI. I think that’s one of the most helpful mental shifts to make. It’s not to say there are no discontinuities at the point where these systems can do better AI research than all of OpenAI. That does feel like some kind of discontinuity. But on the whole, I think thinking about it as a curve is the right framework.
John:
You see AGI as a continuous journey, not something with discrete steps?
Sam:
I mean, there are some steps, but if you zoom out, it’s pretty continuous.
John:
What excites you about what will happen in that continuous journey towards AGI this year?
Sam:
You know, this sounds like an evasive answer, but the thing is that the models will get generally smarter. I think that’s really the special thing. It’s not that we’re going to add this modality, or that modality, or that we’re going to get better at this kind of reasoning, and better at this sort of part of the distribution. It’s that the whole thing is going to get generally smarter across the board, and that is a state… The fact that we’re living through this sort of AI revolution is going to, I think, seem much crazier in the history books than it does right now, and a statement, the fact that we’re like, actually, everything just gets better. The whole model gets smarter. It’s the G that’s improving, that’s amazing.
John:
That’s great. The thing I find fascinating, Sam, is it seems like for most types of knowledge work today, the starting point seems like you start with ChatGPT, it almost feels like the cursor to the computer could be ChatGPT, where for-
Sam:
I think there is something about language as the interface that is going to be deep about how we use computers, and it’ll be a way that we start a lot of our work with computers. It does feel to me like this is a new interface shift on the order of a mouse and a keyboard. The fact that the computer, I think sci-fi got this right, we just want the computers to understand us and do what we want. It won’t be all text or it’ll be all language, there will be some other things too. But yeah, I think this one, this is a significant step.
John:
Yeah. Do you envision these systems getting better at more complex reasoning, like the system two type tasks?
Sam:
Yep, we do. I think that’ll be one of the most important areas for new applications.
John:
Great. It feels like with Google search, we were kind of trained to ask a question, get a quick answer in milliseconds, but with an advanced reasoning system like ChatGPT, you could potentially ask a complex question, the system goes and thinks about it, comes back. Is that how you think about it?
Sam:
Yeah, I think it’ll all work, there’ll be multiple things. Sometimes you’ll want the instant response, sometimes you want a longer, more thoughtful one, and that’s fine.
John:
Are there any particular verticals where you see a greater impact in the rollout of AGI?
Sam:
I mean, yes, but I think it’s missing the point. Again, this is back to the G, the generalized part of intelligence. I think the least interesting thing about what’s going on is, “Oh, is it this vertical, or that one, or should I think about this or that?” This is, again, when you’re living through history, it feels like the newspapers. It’s just like, “All right, this is the thing that happened yesterday, and this is that, and this is that, and there’s this drama here in this industry here.”
And then, at some point, it will get consolidated into a really important chapter in the textbook. And when you zoom out to that level, it won’t be about, “Was it this industry or that one?” And it’ll feel like a much bigger deal than it seems to us at the time, and also way less focused on the details.
John:
Great, great. And when we think about the next level of breakthroughs, the next level of improvements in performance, how much of it do you see as coming from more scale, bigger model, train a larger network for longer, versus algorithmic breakthroughs, like maybe going from transformers to a different architecture?
Sam:
That’s all of those things. Everybody wants the, “Oh, is it this or that?” And I never really get the spirit of that question, but it does seem like people really want it to be, “It’s this,” or, “It’s that.” It’s 200 things that multiply together that we work really hard on each piece of.
John:
That’s great, that’s great. Yeah, I recall you saying that one of the things OpenAI is really good at is accumulating these 200 medium-level things and multiplying it all together to have this one big leap forward. So in terms of the impact of AGI, it still feels like there is a pretty significant gap between what type of impact AGI could have on the economy, on global GDP, versus what’s actually happening today. I feel like one year back, we would’ve probably thought businesses would’ve changed faster, Fortune 500 companies would’ve adopted AI faster. Why do you think that is? What do you see as the gap between value realization and potential?
Sam:
I think the early innings of all technology revolutions are slower than you think they should be, and then it sort of speeds up over time. But, you know, we’re still at the stage where the technology is still very weak. It’s still very brittle. Society has a lot of inertia and hasn’t quite figured out how to adapt it where it works. It’s just going to take a while, give it some time. GPT-4 was released one year ago.
If you study any other major new scientific or technology discovery rollout that you want, give it some time, the impact will be there. And I think it’s also good that… I think if we could build AGI tomorrow, by however you want to define it, you would sort of think society should change an unimaginable amount that next year, and I think it’s good that it probably won’t. It’s good that there’s some inertia. It’s good that people take some time to change. It’ll still feel really fast when we live through it.
John:
Yeah, it’s been super fun. When we speak with these Fortune 500 companies, oftentimes we see that, usually in a month, we do our own version of iterative deployment, it feels like it’s possible to demonstrate some value really, really quickly relative to supervised machine learning, where it would take a few months to assemble the data set and really train a model. When you build on top of GPT-4 or GPT-3.5, it just feels like we are at a point where it is easy to demonstrate value quickly, and then hopefully it’s going to catch on this year. I feel super excited about what’s going to happen this year.
Sam:
If it happens next year or the year after, it’s okay. It will happen.
John:
Absolutely, great. And Turing, Sam, has this platform of three million software engineers, and most of them are using GPT-4 for coding and becoming more productive. What’s your advice for software engineers in the era of AI-assisted software engineering?
Sam:
I think this has got to be the best time to be a software engineer in a very long time. It is truly a time of a new way to work and new frontier. These don’t come along that often. It’s amazing to me how much the experience of writing code has already changed, but I think, in the next couple of years, it’ll be the era maybe that we see AI change things the most, and the tool chain that’ll be available, what it’ll be like to write code.
If you become two times more productive with better tools, that’s one thing, and you can just work twice as fast, or maybe a little bit more. But if you become like 100 times more productive, there is a qualitative change at a point, like that probably, well, less than that, where you can just do things you otherwise wouldn’t have been able to do at all. You can keep a problem in your head, you can work at a [inaudible 00:18:04] abstraction you otherwise just couldn’t have. And the people that adapt to that and really start to work with the new tools will do just amazing things, I think.
John:
That’s great. There’s no better time to be a software engineer in this era, so this is going to be super exciting. And it just feels like the lift is going to be so much more than the 20% or 30% that people have shown in tests, particularly when you think about full CodeGen and using GPT-4 in an iterative loop. John, how are we on time for rapid fire, or do we have… Few minutes? Great. So go to one other thing, what’s the aspect of GPT-4’s impact on the world that you are most excited about?
Sam:
Probably the biggest one is just that we got the world to take advanced AI seriously, and we got the world to take that seriously before we’re really in the thick of it. I think time for the world to prepare for our institutions, and people and society to decide how we want this to go, to the degree we can make those decisions, I think that’s really important. I think springing AGI on the world all at once would’ve been quite challenging, and for a while, we tried different things to get the world to care, and finally, we did. So I think that’s the impact we’re most proud of.
John:
So I feel like one big impact of AI is amplifying human productivity and giving people leverage. Do you envision a future where you and I could have virtual or physical avatars that are operating at scale? Maybe while the two of us are talking, there’s a Sam robot somewhere else, having another interview in person with 100 other robots having automated interviews with people?
Sam:
I don’t know, maybe.
John:
What do you see as the biggest blocker to a future like that? How can we get there faster?
Sam:
I’m not sure I want 100 avatars of me running around, I don’t know. I think the future is going to feel like it’s coming plenty fast. Even with my earlier comments about the inertia being good, I think it’s going to feel like it’s coming fast, and without that inertia, we would just be in a really tough place. So I don’t think we need to do more to make… I mean, I think we all need to keep working hard. I don’t think the world gets better on its own, but I think we’re going to do that, and I think we’re going to get a future coming pretty fast.
John:
Great, great. One thing I found really inspiring about OpenAI’s mission is also how these advanced AI systems can speed up the pace of scientific progress itself, right?
Sam:
That’s the thing that I am personally, just as someone who wants to see the world get better, that’s the thing I’m personally most excited about. I think AI’s going to be amazing in all these different ways, but when we can start speeding up the rate of knowledge discovery, and use that to cure diseases, or address climate change, or teach everybody on Earth in a much better way than we know how to do today, I’m a believer that science and technology, it’s almost the only way, at least the super majority, of how we get sustainable economic growth, and that sustainable economic growth is a driver of so much of what we want. But that’s the thing I’m most excited for. I think it’ll be more transformative than we realize.
John:
Great, great. And do you see a future where OpenAI does more work on robotics? You have a rich history of doing work in robotics.
Sam:
Yeah, we always thought robotics is cool. We still think robotics is cool. We stopped working on robotics, really, for two reasons. One is robots were hard for the wrong reasons. It was like the physical things break a lot and they’re slow. It wasn’t hard because it helped us discover, do good like ML science, and that was what the most important thing to do was. And the other is we frankly just got language models to work and said, “Wow, this is a really big deal. We want to focus our effort here,” but we’d like to get back to it someday.
John:
Great, great. And so we’ll transition very quickly to a rapid fire round. We have a few quick questions. Sam, in one word, describe the future of AGI.
Sam:
Capable.
John:
Capable. Okay, what’s the most inspiring piece of tech from your childhood?
Sam:
A tie between that first iconic iMac and a Motorola StarTAC, my parents’ cell phone that I thought was so cool.
John:
Great. So if you could finish the sentence, “Five years from now, we will…”
Sam:
I have no idea.
John:
Okay, great, that’s great. Thank you, Sam. Now we’ll get the audience involved and field a round of questions from them. All right. Where do you see open source going and is OpenAI planning on releasing models that can be hosted internally?
Sam:
I think there’s a super important place for open source in the ecosystem. I continue to root for its success. What do you mean by “host models internally”?
John:
I imagine an enterprise might want to run a hosted-
Sam:
Oh, oh. Yeah, I’m not opposed. Not like an immediate…
John:
Whoever asked that question, you should use Turing. We’ll do that. Okay, great. We will implement OpenAI’s models and do that. So what areas out of IT or software development do you see AI impacting the most, automotive manufacturing? I think Sam’s answer is it’s going to be “everything”. What are your views on the role of human intelligence as we get closer to AGI?
Sam:
Great question, and I think there will be more answers than the one I’m about to give. But one thing that I’ll point out is humans seem very good at knowing what other humans want. Humans also seem to care a lot about other humans. The example that I like to give for this, because I always love to go back and read contemporaneous accounts of a new technology rolling out. So it’s interesting to watch, to read the stuff about the Industrial Revolution, when that happened. But the example I was going to give here is when Deep Blue beat Garry Kasparov, which was kind of one of the first moments of real AI in the public consciousness.
And I think we forget what a big deal that was, but at the time, chess, that was the great intellectual pursuit. “If a computer could be a human in chess, what does that mean?” It was just this huge deal, if you go read the contemporaneous accounts. And the prediction at the time was that chess was totally over, that was it. That was the end of chess, and it was maybe the end of humanity, but it was at least the end of chess. And if we fast-forward, the decades since, chess has never been more popular than right now. Chess has also, and this is maybe just mostly a factor of the internet, but it’s never been popular to watch then right now. And no one watches two AIs play each other. And if you use AI to cheat, that’s a really big deal.
So I think the predictions of where we’re still going to really value humans, and human touch, and human creativity, and curation, that’s going to surprise us. When I read a book that I really love, the first thing I do is I want to know all about the author’s life story. I really care about the person behind that. When I use a product that I think is really beautifully designed, the first thing I try to do is figure out who that person was, and I want to understand them, and I care about them. I think there are going to be a lot of versions of that that we underestimate.
John:
Yeah, great. What do you think is the best way to safely deploy existing imperfect models in areas that require reliable answers, like self-driving and medicine?
Sam:
Carefully and slowly, and in most cases, with a human in the loop, until we’re confident that we’re at a degree of reliability that’s acceptable. A thing that I think about, I would just like to know what is the multiple on safety that a self-driving AI has to be better than a human for society to accept that? Maybe it’s 10, I think it’s more than 10, and I think that’s going to apply in a lot of other industries.
Humans are somewhat forgiving of humans making mistakes, they’re very unforgiving of computers making mistakes. And so this is this very interesting question, if you had a life and death medical decision, and you could have an AI make it with some percent error rate, but it was going to get stuff wrong sometimes, and if you could have a human make it with a higher percent error rate, what does that delta have to be before you say, “I will hand this decision over to the AI?” “Really high,” I think is the answer for me, and that’s totally irrational
John:
What comes after LLMs?
Sam:
Again, I think that it’s really fun to debate, “Is it going to be this architecture or that architecture, and are we going to find… Is it going to be more about the bigger computer or this, and this thing we’re going to do on top of LLM, or we’re going to do our pre-training differently,” or whatever, whatever. I so encourage you to zoom out from that. Again, I love thinking about that, and I have to urge, encourage myself to zoom back from that. It’s just going to get smarter every year from here. We’re not going to hit a wall. We’ll change all kinds of things. I mean, maybe millennia in the future we hit a wall, but it’s just going to keep getting smarter.
John:
You mentioned the path to AGI will be a power struggle, but it seems like technical efforts are still the majority of activities for progress. Will that hold true, going forward?
Sam:
No one knows. There are a lot of strong opinions, always, about, “It’s going to go this way or that way,” or this is, “I know what the government’s going to do,” or, “This is what’ll happen here.” The people that like to prognosticate about AI, particularly about AI safety, but AI in general, really have a lot of strong opinions, I think that don’t take into account what a complex system the world is, and also the fact that it’s just very branching probabilities.
And so, there are all these ways things could go. No one even knows what the right probabilities are, but also there are probabilities, and they’re path dependent in all these different ways. So I think the way to manage through something like that is make each individual decision as low stakes as you can, and have a very tight feedback loop, and iterate. I think that is the strategy that will work.
John:
What’s your advice for folks working on AI research?
Sam:
Whoever asked that, is the question, what area to push on, how to be a good researcher in general? That’s a great question, but it could mean many things.
John:
I think we’ll interpret that as what area to push on, unless somebody wants to…
Sam:
What area to push on?
John:
Yeah.
Sam:
I don’t know, if whoever asked that, if you want to email me and tell me what you’re interested in, and what you’ve worked on, I can try to give you custom advice. If I say the same… That’s a hard question to answer. It’s one of my favorite questions to try to talk to someone about, what research they specifically should work on. But it’s hard, in the general case.
John:
Yeah. So companies that want to use GenAI but aren’t standing up their own teams, where do you see leaders emerging who can help us integrate and adopt these systems after buying ChatGPT and these other models? I don’t know if that’s well formed, let’s skip that.
Sam:
I think one of the cool things about this is it gets easier as the models get better. This is different than some other kinds of technology, but at some point, you can just ask the model, “How should I integrate you into my product?” Right? But in the meantime, you’ll be able to just sort of throw in more data, into bigger contexts. You’ll be able to give less specific prompts and still get what you want. Most of the time, it’ll be way more robust. So I think this is going to be different than the shape of a lot of other technologies we’ve seen in that it gets easier and easier with each model crank. You need less expertise.
John:
Great, great. So one last question, what aspects of deploying AI do you believe are currently under-discussed or under-appreciated today, and yet pivotal to its success?
Sam:
I mean, I think the stuff still mostly doesn’t work, right? So to build a system that works in spite of all of the current flaws, to find the limited area, and kind of the machinery you have to build around something, I think that’s still under discussed. These systems are unbelievable at creating compelling demos, and still quite difficult to make a really great, robust product out of. And I think that is even for people that kind of intellectually know that you don’t want to believe it, or we don’t want to believe it, and so that doesn’t get discussed enough.
However, this is maybe a good point to close on. I think startups building on top of AI are very much in two different categories. They’re the ones that are betting against the models getting better, implicitly, and the ones that are betting that the models will get better. And if you’re building against the models getting better, you put a lot of effort into building something that takes the brittleness and makes it a little easier to use, a little more likely to work. You can work around some of the limitations. And then we go make GPT-5, and it does all of those things and more really well. And then you’re like, “Hmm, that is sad.”
The other category are companies, or products, or services that depend on the model getting better, and that benefit a great deal from the model getting better. Say, “Okay, here’s this thing. It is immigrating intelligence in a new way, into this interesting vertical or whatever. And man, I wish the model were smarter. If the model were smarter, it would just be better. I’d have 10 times more customers.” And then, you’re thrilled when GPT-5 comes out. It seems to me, in a vacuum, that most of the world should want to work on that second category, but in practice, it’s very hard for us to convince people to do that. And then every time we put out a new model, there’s just all of the “OpenAI killed my startup” meme going crazy. But the models are going to keep getting better, and you should build something that is aligned with that happening.
John:
Note for everyone, don’t bet against the models not getting better. They are going to get better. Sam, this was wonderful. And for all those in the audience who are excited about working on making the models get better, if you could email agiicons@turing.com, like if you’d like to work on these problems with OpenAI, we’ll collect all of that and send that to the couriers, send that to the OpenAI team.
Sam:
Awesome.
John:
Great, and any parting words of advice for the researchers in the audience that are excited to work on AGI?
Sam:
I mean, you should definitely work on it. Yeah, reach out to us. We’d be excited to talk, I guess.
John:
Great, great. Wonderful, thank you.
Sam:
Thank you.